10 Reasons Humans are Terrible at Hiring (and How AI Can Help)
Last year, Amazon shuttered an algorithm the team was developing because it realized it was biased. The algorithm was developed by the company’s engineers to look at high-performing employee profiles and then select the right candidates from the firm’s resume database. The problem? The algorithm favored men in its selection process.
When this story hit the news, it became a rallying cry for those that do not believe in the value of AI for recruiting and hiring. However, as someone who’s written the book on the subject, I think it’s a great example of what a good algorithm can do if it’s developed, trained, and deployed in an unbiased way.
The issue in this story isn’t that an algorithm was picking men. That’s what Amazon trained it to do! The core issue is that Amazon has historically hired, promoted, and given good reviews to men more often than women. So when the algorithm is trained on that data set, it can only come to the conclusion that men are better than women (even if every decision the company made in its hiring and performance decisions was biased, as the list below demonstrates).
Fundamental (Mental) Bias
It’s really easy today to get caught up in the headlines:
- AI is biased!
- Humanity doesn’t stand a chance!
- Algorithms are going to kill us all!
The truth is that artificial intelligence, in its current form, is ideally poised to help minimize some of the very real biases that humans have that limit our ability to hire effectively.
Below are 10 of the common biases that affect human decision-making in every aspect of life, but in this scenario, the context is all around hiring and candidate selection.
- Dunning-Kreuger Effect: we think we are better than we really are at making hiring choices, even though we’re just as bad as everyone else.
Hiring translation: I am a really great interviewer. I almost always make the right choice of candidates. - Confirmation Bias: we want to believe ideas that confirm what we think to be true.
Hiring translation: “this candidate went to the BEST school, so they have all the BEST answers to our interview questions” - Self-serving Bias: others cause our problems, but we cause our own wins.
Hiring translation: “Jerry told me to hire Maria but she didn’t work out; however, I hired Jamie and she was a great choice.” In this example the hiring manager may have overtly or subconsciously given Maria signals that she wasn’t wanted, and she left because of them. Those same signals weren’t given to Jamie because she was actually the desired candidate. - Self-handicapping: we could make the right choice but we have an excuse in hand just in case.
Hiring translation: “I just knew Elvis wouldn’t work out for us. I wanted to wait another month to pick but someone else made me choose a candidate before I was ready. We were rushed to hire.” This is all about setting up an “out” or an excuse in case it doesn’t work as expected. - Optimism/pessimism or headwinds/tailwinds: we overestimate likelihood of good things and underestimate bad things; alternatively, we overestimate the things that cause us to fail (headwinds) and underestimate the tailwinds that set us up for success.
Hiring translation: I hope we can hire a great crop of candidates this college recruiting season, but we are missing a recruiter and just don’t have the relationships we used to with candidates at key universities. (In this example, the company overestimates the negative headwinds and underestimates the longstanding relationship with the university, the value of the right technology to support the process, and other positive aspects that would have set them up for success). - Sunk Cost: we cling to things we already have, even if they hold little to no present value.
Hiring translation: I know Bob isn’t really working out, but it’s just too much hassle to hire someone else so let’s hold onto him for a while longer. *Note: I call this the red button test. If you had a red button to replace that person immediately, would you press it? If so, it’s time to replace them because sunk cost isn’t a reason to keep something of little/no value. - Decline Bias: a belief that things are worse or harder today than they were in the past.
Hiring translation: there is no good talent today. It’s harder to hire than ever before. THE WAR FOR TALENT. Et cetera. - Backfire Effect: we become more convinced and double down after we are challenged on a position.
Hiring translation: I know she may not be the best candidate but she is the best one we can find. We really just need to hire her as soon as possible. - Anchoring: the first thing we see influences how we evaluate everything else.
Hiring translation: That first candidate was really outgoing and a great conversationalist. Lots of fun. None of the other candidates were really as talkative as that first one… - Halo/horn Effect: happens when one attribute or trait overshadows others in a positive (halo) or negative (horn) manner.
Hiring translation (halo): that candidate did an amazing job on the assessment. I think she would be great for any job we have available right now.
Hiring translation (horn): wow, that last interview was rough because the guy was a smoker. I didn’t hear anything he said in response to the questions because I couldn’t breathe.
Admit It: We’re Bad at Selection
The data show that the common ways we interview and many of the methods companies use to rank candidates (university, college grades, or other demographic data) are highly unreliable statistically. Translation: they are terrible as a gauge for whether someone can do a job or not.
Instead we should rely on more reliable types of data sources, such as job samples (let someone try the job before they are hired to do the job), assessments, or structured/regimented interviews. If we can use these more predictive types of data, we CAN make better hires and improve quality of hire. And, interestingly enough, AI is positioned to do just that.
AI can help to mitigate or eliminate these types of biases if developed with a diverse team and trained on an unbiased, comprehensive data model. At a minimum, AI can help to limit the times when humans have to make decisions in the absence of data, as the list of biases above prove that we can’t make accurate, rational decisions (and sometimes we can’t even if we DO have data).
Which of these biases is a problem for your team? For you? How can you overcome them?
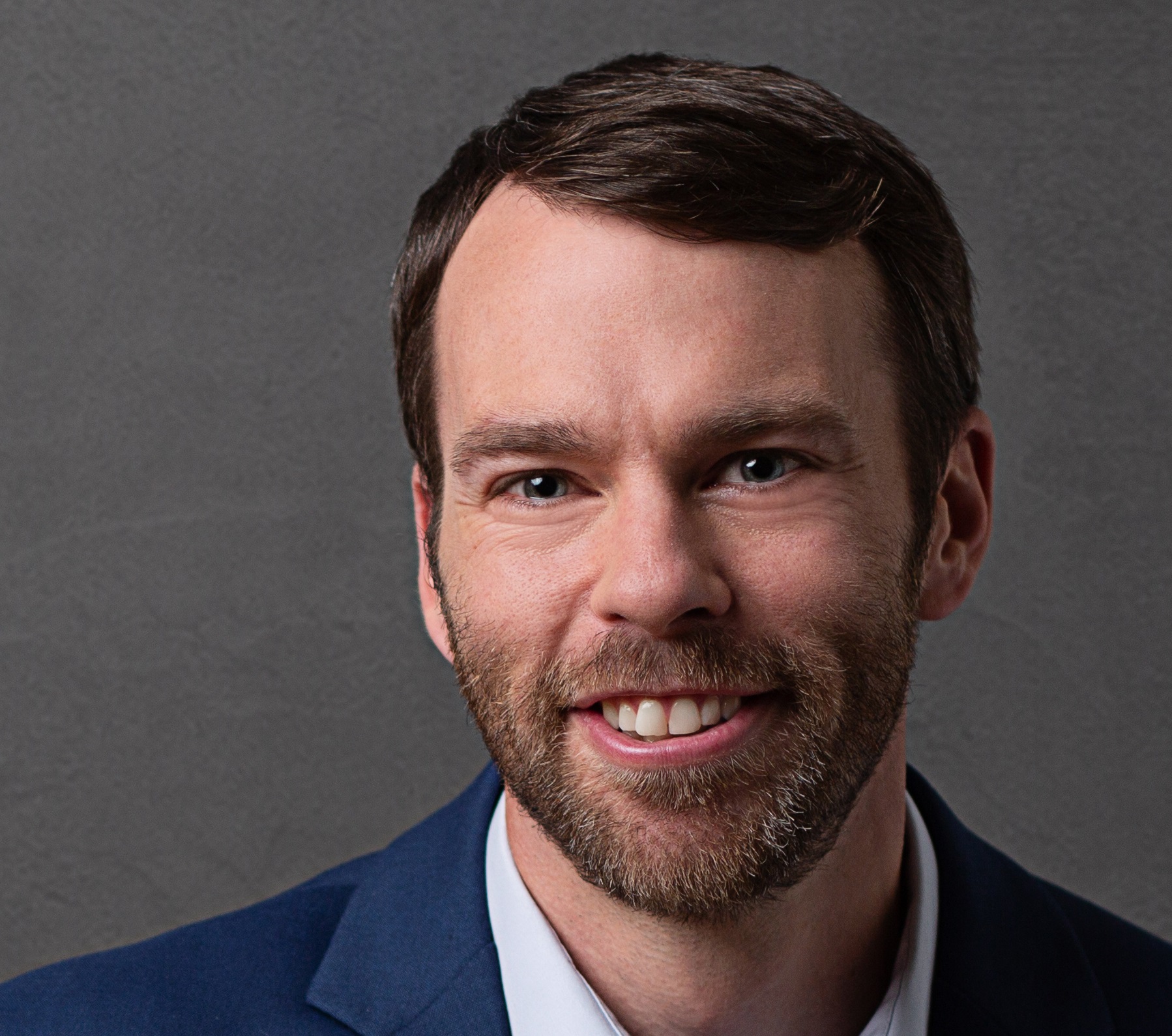
Ben Eubanks is the Chief Research Officer at Lighthouse Research & Advisory. He is an author, speaker, and researcher with a passion for telling stories and making complex topics easy to understand.
His latest book Talent Scarcity answers the question every business leader has asked in recent years: “Where are all the people, and how do we get them back to work?” It shares practical and strategic recruiting and retention ideas and case studies for every employer.
His first book, Artificial Intelligence for HR, is the world’s most-cited resource on AI applications for hiring, development, and employee experience.
Ben has more than 10 years of experience both as an HR/recruiting executive as well as a researcher on workplace topics. His work is practical, relevant, and valued by practitioners from F100 firms to SMB organizations across the globe.
He has spoken to tens of thousands of HR professionals across the globe and enjoys sharing about technology, talent practices, and more. His speaking credits include the SHRM Annual Conference, Seminarium International, PeopleMatters Dubai and India, and over 100 other notable events.