#AI in #Learning: 8 Learning Content Recommendation Signals for Personalized Experiences
AI is penetrating into every aspect of HCM, including learning and development. In many briefings with technology providers I’m hearing the same thing over and over again: we designed this experience to be like the Netflix of learning content.
That’s a nice sentiment, but Netflix is an entertainment streaming service with highly personalized experiences for users. You’re offering learning content, and it’s often not that tailored to the individual. So it’s not quite the same, for obvious reasons. However, by looking at some of the ways we recommend training, we can improve the learning experience without creating an overwhelming interface for learners.
Today, there are an incredible number of options for learning content, whether inside or outside the organization. And curation is quickly becoming the name of the game for learning leaders. The challenge is in how to curate the content to deliver the most personalized, tailored experience for users.
Today’s algorithms can factor in a ton of signals to determine what recommendations the machine will make. For example, Amazon has had a patent for years that allows it to anticipate the needs of users based on a significant amount of user data and interactions. Below is an overview of some common and not-so-common signals that, when matched with AI, can create a more personalized learning experience for all of your people.
8 Learning Content Recommendation Signals
- User preferences – by far the easiest to set up in someone’s profile, user preferences can be tied to job or role. For example, managers take managerial training. This can also include other defaults or user-defined preferences such as content about specific aspirational topics or skill areas of focus.
- Consumption by similar people – by looking at what similar people take within the LMS or LXP (learning experience platform), systems can recommend training that you might also be interested in that share your current role or interests. If other design specialists are taking the UX course, the system can recommend that you also take the UX course if you’re also a design specialist.
- Consumption by people in my preferred role/career path – this takes the point above a step further. If you’re currently a marketing associate but want to be a marketing manager, the system can highlight courses being taken by those at a higher level to help you see what kinds of learning content you need to succeed in that preferred or aspirational role or career path.
- Views (popularity)– recommending popular content isn’t a bad move, especially if you’re trying to get a critical mass of traffic onto the learning platform, but this should not be the only indicator of what content to consume next. We’ve all been sucked into the funny work video that goes around via email and IM at work–those are popular but don’t necessarily impact performance.
- Star ratings and comments (value) – unlike raw view counts for popularity, star ratings and comments can offer a deeper layer of insight into the quality and value of content. Star ratings, thumbs up/down voting, or other simple measures give a feedback loop about whether content is valuable or not, and comments can offer deeper insights into the specific value or feedback points from users. Note: the team at onQ actually has a way to do this inside video assets where users can comment and create asynchronous social learning conversations on videos within a learning library. The algorithm then shows where users are engaged, disengaged, or confused in 10-second increments within a video. Very interesting.
For many learning systems, these first five components are either standard or becoming standard. But what’s next on the horizon? Consider the following items we’ve seen other companies following in a consumer context that might help us to drive more consumption of learning content.
- Time of day, week, year – in a recent interview with Wired magazine, the product team at Netflix talked about how its algorithm selects content for users. One surprising component was time of day. If users are looking at content late at night, the system is more likely to recommend partially consumed content (finishing off earlier views) than new, unwatched content. This could be extrapolated for learning purposes. If someone is logged in from a public wifi, they may be better suited to shorter content (potentially commuting or on travel) whereas logging in from work when their calendar has three hours available might signify the potential for consuming longer-form content. On a weekly basis, this might also flow around work. On an annual basis, users may want deeper dives early in the year to create new competencies but later in the year may be settled in and looking to hone or refine established capabilities.
- Title performance in search vs. consumption – research from Groupon’s data science team shows that there is a science behind what we open and read, and this has direct translations to what people open and examine from a learning context. What Groupon found was that by examining the performance of what had encouraged opens and clickthroughs in the past, the team could target those same types of terms and syntax to create higher engagement with the audience. In learning, we can measure this by modifying the titles and descriptions we define and then measuring that against consumption patterns. Titles may change open rates but may not affect completions, and descriptions may or may not contribute, but without considering them and measuring the impact, it’s hard to say. Netflix and other tech firms use A/B tests to see which content is consumed more often, then the highest converting option is scaled across the platform for all users to ensure the best performance.
- Job performance/productivity/output – It’s easy for a Netflix or an Amazon to see what is working or not: subscriber counts support their approach. At work, we need to get better at this, though. One interesting approach I recently saw was IBM’s Your Learning LXP that targets key skills across the organization, creating a powerful set of reports that leaders can use to not only see training volume and demographics but also impact.
These are some of the signals we’ve run across in our research. Though this isn’t an exhaustive list, it helps to highlight how artificial intelligence technologies are supporting areas like learning and development through better content curation.
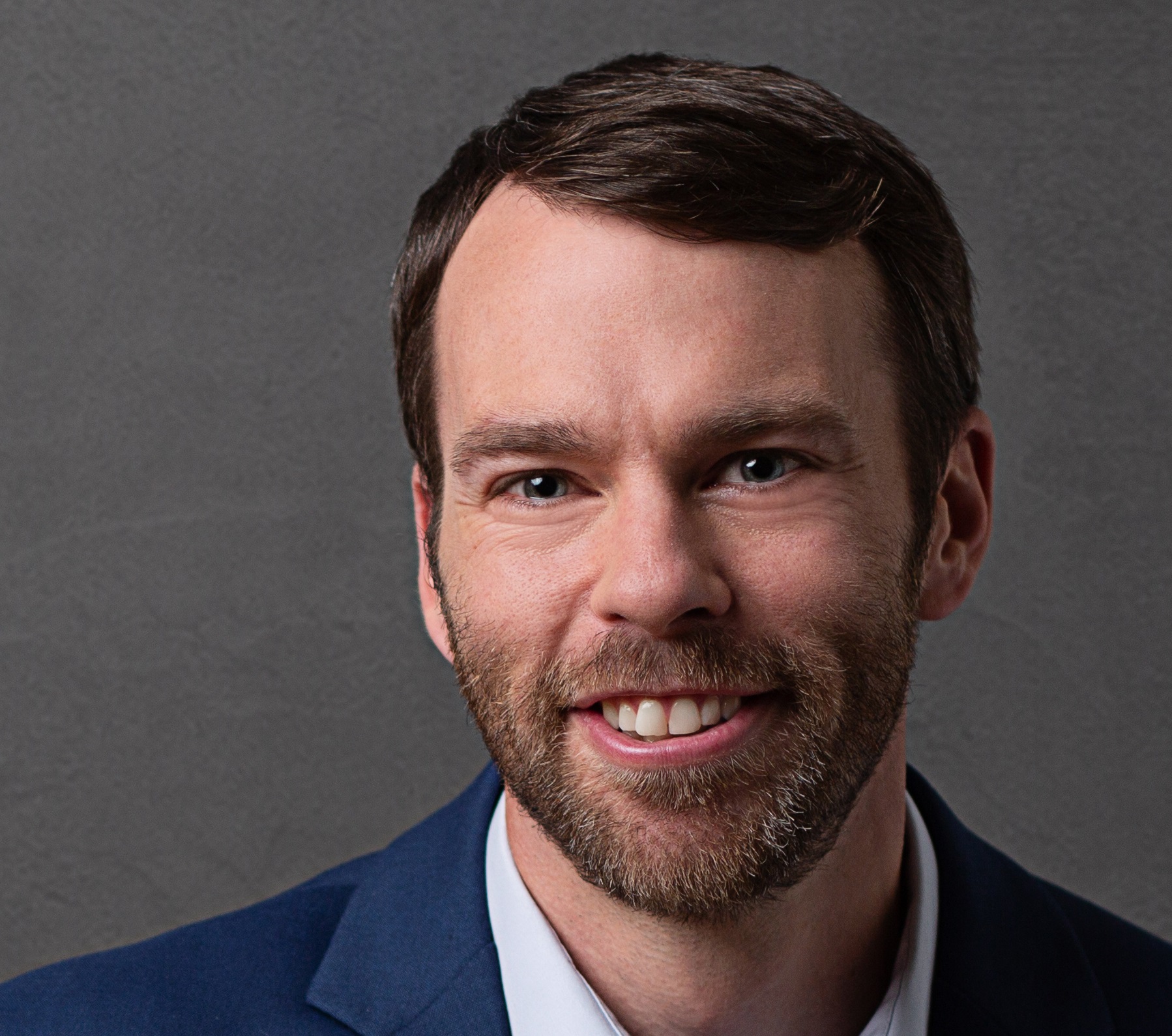
Ben Eubanks is the Chief Research Officer at Lighthouse Research & Advisory. He is an author, speaker, and researcher with a passion for telling stories and making complex topics easy to understand.
His latest book Talent Scarcity answers the question every business leader has asked in recent years: “Where are all the people, and how do we get them back to work?” It shares practical and strategic recruiting and retention ideas and case studies for every employer.
His first book, Artificial Intelligence for HR, is the world’s most-cited resource on AI applications for hiring, development, and employee experience.
Ben has more than 10 years of experience both as an HR/recruiting executive as well as a researcher on workplace topics. His work is practical, relevant, and valued by practitioners from F100 firms to SMB organizations across the globe.
He has spoken to tens of thousands of HR professionals across the globe and enjoys sharing about technology, talent practices, and more. His speaking credits include the SHRM Annual Conference, Seminarium International, PeopleMatters Dubai and India, and over 100 other notable events.